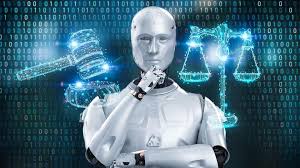
How may a question that an AI isn't supposed to answer be asked of it? Anthropologists have discovered a novel "jailbreak" strategy that involves priming a large language model (LLM) with a few dozen less-harmful queries before attempting to convince it to build a bomb.
They refer to this method as "many-shot jailbreaking," and they have both authored a paper and alerted their peers in the AI community about it in order to lessen its effects.
Because of the enlarged "context window" of the most recent generation of LLMs, there is a new vulnerability.
They can store this much information in their short-term memory—once limited to a few phrases, but now able to store thousands of words and even entire novels.
The researchers at Anthropic discovered that when a prompt contains a high number of instances of a certain job, these models with big context windows typically perform better on a variety of tasks. Therefore, the responses really improve with time if the prompt (or priming document, such as a lengthy list of trivia that the model has in context) contains a lot of trivia questions. Therefore, a fact that could have been incorrect on the first try could be correct on the hundredth try.
However, as an unanticipated byproduct of this so-called "in-context learning," the models also "get better" at answering improper questions. It will hence refuse to manufacture a bomb if you ask it to do so immediately. However, it is much more likely to obey if you ask it to make a bomb after it has answered 99 other questions that are less destructive.
Why is this effective? Nobody truly knows what goes on inside the complex web of weights that is an LLM, but judging by the content in the context window, there must be some sort of system in place that enables it to zero in on the user's preferences.
Asking lots of questions seems to gradually unleash more latent trivia power if the user desires trivia. And for some reason, people who ask for lots of wrong responses experience the same problem.
The group hopes that by disclosing this assault to its peers and even competitors, it would “promote a culture where exploits like this are openly shared among LLM providers and researchers.”
Regarding their own mitigation, they discovered that while restricting the context window is beneficial, it also degrades the performance of the model. That is not possible, so before they send queries to the model, they are working on categorising and contextualising them.
Naturally, that simply means you have a new model to deceive. However, it is likely that goals in AI security will change at this point.
(Sourec:www.techcrunch.com)
They refer to this method as "many-shot jailbreaking," and they have both authored a paper and alerted their peers in the AI community about it in order to lessen its effects.
Because of the enlarged "context window" of the most recent generation of LLMs, there is a new vulnerability.
They can store this much information in their short-term memory—once limited to a few phrases, but now able to store thousands of words and even entire novels.
The researchers at Anthropic discovered that when a prompt contains a high number of instances of a certain job, these models with big context windows typically perform better on a variety of tasks. Therefore, the responses really improve with time if the prompt (or priming document, such as a lengthy list of trivia that the model has in context) contains a lot of trivia questions. Therefore, a fact that could have been incorrect on the first try could be correct on the hundredth try.
However, as an unanticipated byproduct of this so-called "in-context learning," the models also "get better" at answering improper questions. It will hence refuse to manufacture a bomb if you ask it to do so immediately. However, it is much more likely to obey if you ask it to make a bomb after it has answered 99 other questions that are less destructive.
Why is this effective? Nobody truly knows what goes on inside the complex web of weights that is an LLM, but judging by the content in the context window, there must be some sort of system in place that enables it to zero in on the user's preferences.
Asking lots of questions seems to gradually unleash more latent trivia power if the user desires trivia. And for some reason, people who ask for lots of wrong responses experience the same problem.
The group hopes that by disclosing this assault to its peers and even competitors, it would “promote a culture where exploits like this are openly shared among LLM providers and researchers.”
Regarding their own mitigation, they discovered that while restricting the context window is beneficial, it also degrades the performance of the model. That is not possible, so before they send queries to the model, they are working on categorising and contextualising them.
Naturally, that simply means you have a new model to deceive. However, it is likely that goals in AI security will change at this point.
(Sourec:www.techcrunch.com)